Julius O. Smith III
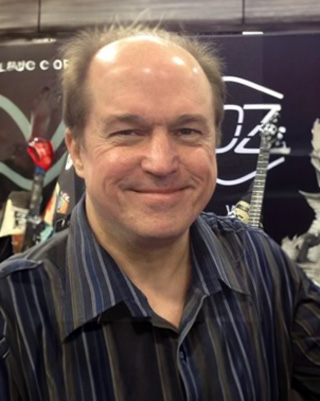
Julius O. Smith is a research engineer, educator, and musician devoted primarily to developing new technologies for music and audio signal processing. He received the B.S.E.E. degree from Rice University in 1975 (Control, Circuits, and Communication), and the M.S. and Ph.D. degrees in E.E. from Stanford University, in 1978 and 1983, respectively. For his MS/EE, he focused largely on statistical signal processing. His Ph.D. research was devoted to improved methods for digital filter design and system identification applied to music and audio systems, particularly the violin.
From 1975 to 1977 he worked in the Signal Processing Department at ESL, Sunnyvale, CA, on systems for digital communications. From 1982 to 1986 he was with the Adaptive Systems Department at Systems Control Technology, Palo Alto, CA, where he worked in the areas of adaptive filtering and spectral estimation. From 1986 to 1991 he was employed at NeXT Computer, Inc., responsible for sound, music, and signal processing software for the NeXT computer workstation.
After NeXT, he became a Professor at the Center for Computer Research in Music and Acoustics (CCRMA) at Stanford, with a courtesy appointment in EE, teaching courses and pursuing/supervising research related to signal processing techniques applied to music and audio systems. At varying part-time levels, he was a founding consultant for Staccato Systems, Shazam Inc., and moForte Inc. He is presently a Professor Emeritus of Music and by courtesy Electrical Engineering at Stanford, and a perennial consultant for moForte Inc. and a few others. For more information, see http://ccrma.stanford.edu/~jos/.
Signal Processing Formulations of Sequence Models
Status: Available NowToday’s sequence models (such as large language models) in machine learning (AI) arose from a blend of principle-based design and empirical discovery, spanning several fields. This talk describes how many of the main ideas could have emerged from an elementary signal-processing approach. This viewpoint offers some features:
- Signal processing folks can quickly learn what is happening in a motivated way
- Machine-learning experts might benefit from signal-processing insights
- Obvious suggestions for things to try next naturally arise